Three Ways ENVI Plays Well with ArcGIS® for Server
Anonym
In a previous blog post I spoke about the interoperability between ENVI and ArcGIS® for Desktop. This included things like data format support, drag and drop of data between interfaces, single-click push of ENVI derived data to ArcGIS, and the ability to run ENVI analytics from directly within the ArcGIS desktop software. Now I'd like to go over some of the ways that ENVI plays well with ArcGIS for Server, and how users can utilize this interoperability to streamline workflows.
Accessing ArcGIS for Server from ENVI
The first scenario I'd like to look at is using ENVI to directly access and analyze an Image Service being served from ArcGIS for Server. ENVI has full support for the image service specification, and can easily consume and analyze services from ArcGIS for Server.
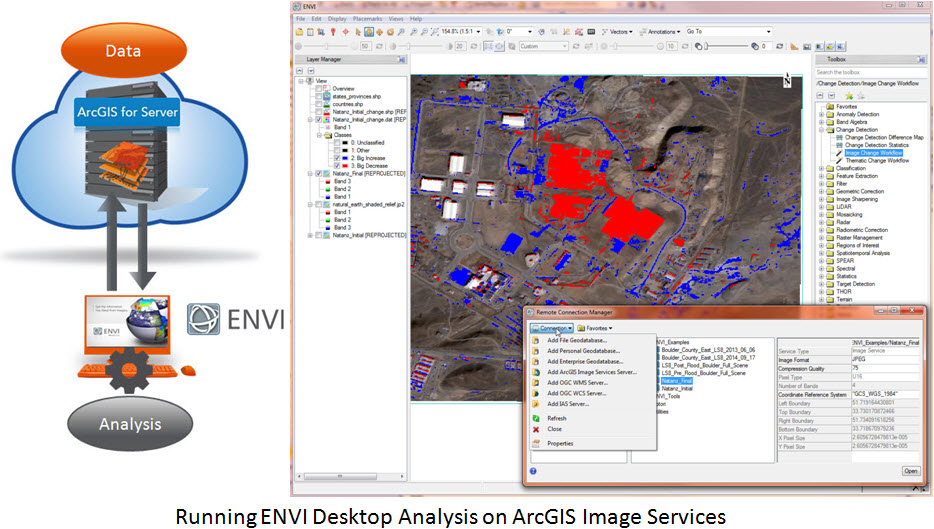
Access is made using the ENVI Remote Connection Manager, which allows you to connect to your ArcGIS for Server and access the data contained there. Once you've connected to your dataset(s), you can use any of the analysis tools available in ENVI to analyze the data behind the service, bringing results local for further analysis. Once the data has been accessed and analyzed, you can then push your derived results over to ArcGIS desktop using the afore-mentioned desktop interoperability, and publish those results back to your ArcGIS for Server for dissemination. This enables any image service with the full suite of analysis tools available in ENVI, and provides a quick method for getting those results back into ArcGIS for Server and shared with the rest of your organization.
Running ENVI Geoprocessing Services through ArcGIS for Server
The second scenario I'd like to explore is running ENVI analytic functionality from the ArcGIS desktop via an ArcGIS for Server instance. In this case ENVI for ArcGIS - Services Edition has been installed alongside ArcGIS for Server, exposing the ENVI functionality as an ArcGIS Geoprocessing Service. ArcGIS can then access not only the data from the server, but can also run analysis using server-based ENVI analytic functions on server-based or local data.
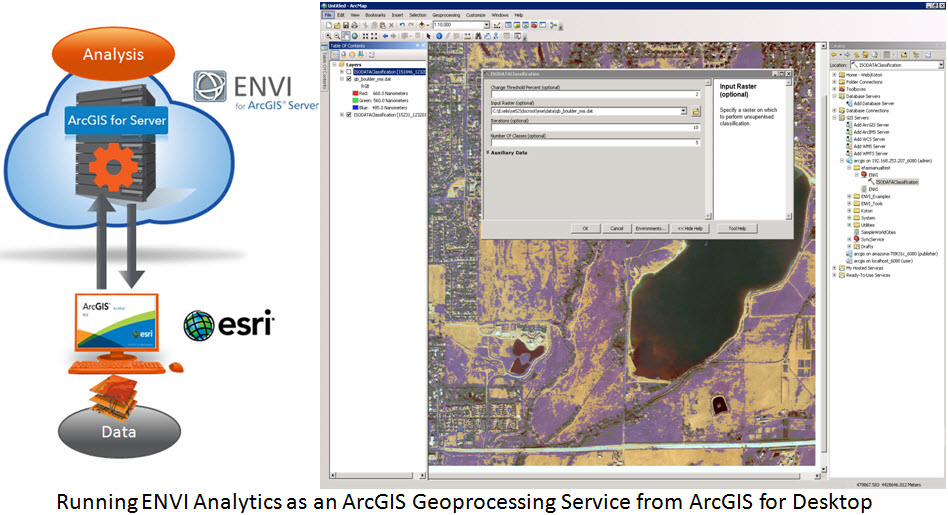
This makes it much easier for developers to centralize the location of their custom analysis while easily exposing that utility across the organization. Analysts can now run ENVI and ArcGIS functionality within the familiar desktop interface and even chain together the different processes into repeatable custom functions using the ArcGIS Model Builder.
Running ENVI Functionality on an ArcGIS Image Service from a Thin Client
The final scenario I'd like to examine is one where neither the data nor the analyses are co-located. In this scenario a thin client such as a webpage designed with the ArcGIS JavaScript API leverages data from an ArcGIS for Server instance along with analysis functionality from an ENVI Services Engine instance. The user makes a request to the ENVI Services Engine, which queries the ArcGIS for Server instance, retrieves the correct dataset and extent, and performs the requested analysis on it. The final derived product can then be made available for download while a visual representation of the analysis is pushed back to the client.
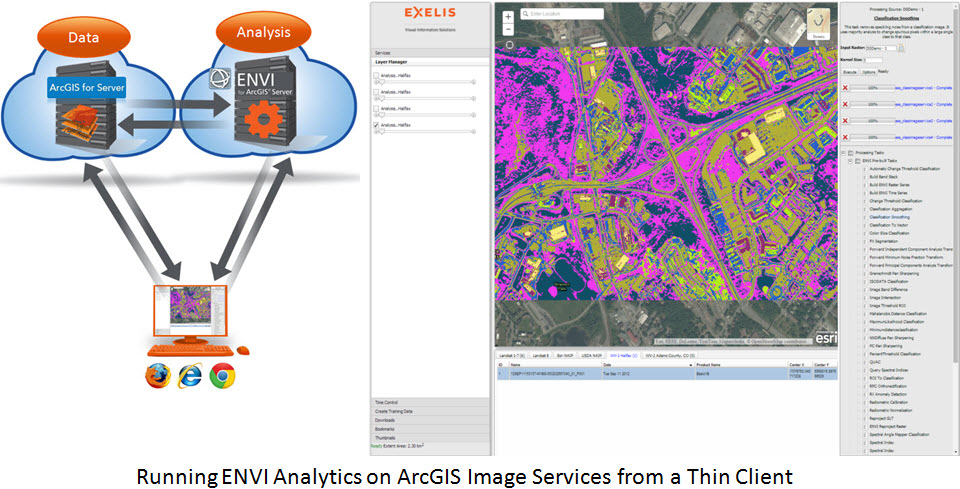
This allows users with low bandwidth to consume advanced analytics on large datasets without having to move data around or have massive computing power on their device. Web pages can be made to consume only the data and analysis that a specific user, or community, is interested in, creating very friendly user-interface experiences for the non-traditional consumer of GIS. It also showcases how standards-based cloud architecture can be designed to consume remote data and analytics from any web enabled device.
These are just three ways that ENVI and ArcGIS for Server can be used to streamline data and analysis workflows within an organization. With new development going into the interoperability between IDL and Python, soon building custom code that leverages the best functionality from both the ArcGIS and ENVI environments will be easier than ever. What do you think? How do you see cloud-based analysis evolving in the future?
*All images in this blog post are used courtesy of DigitalGlobe™ Inc.