Using LiDAR & Deep Learning for Railroad Maintenance and Asset Management
Anonym
As LiDAR sensors continue to evolve, they are now finding themselves in spaces that were traditionally using only Imagery sensors due to the cost to collect. These LiDAR sensors are now small enough to be flown on a DJI PRO or compact enough to be quickly coupled to the front of a Locomotive and collecting as a train takes its normal route. This opens a new possibility of analyzing vegetation encroachment along a rail corridor from the rail center out at ground level, detailed visualizations with clearance in tunnels, and a new means of being able to manage assets and their elevations.
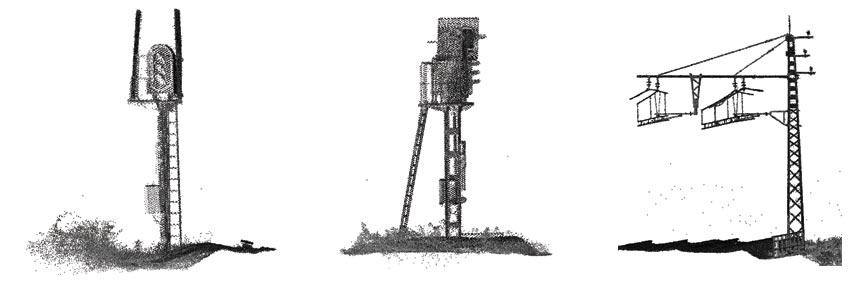
Using our years of vast knowledge of Deep Learning, the team at NV5 Geospatial setout to create a model that would allow us to automatically identify assets along this specific line like power poles, light signals, cabinets, and other assets tied to signaling. As the classifier ran through the dataset it allowed us to build a heatmap of where the assets were along the rail corridor, with a high accuracy of location:
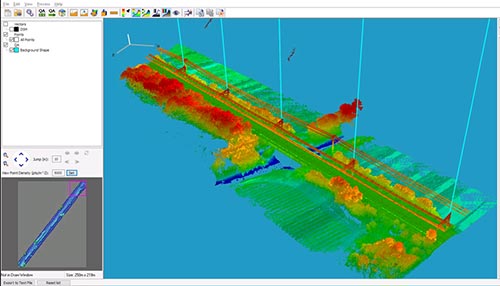
From the heatmap we create our Shape files which are displayed back in the original dataset where we can visually see the accuracy of the points:
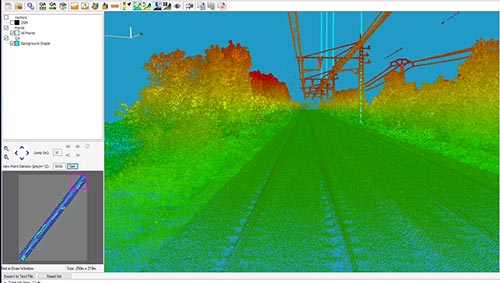
Along with visualizing these assets back in the dataset, we colored our points by height, which provides a look into not only the asset height but features along the corridor like trees that me may be a cause for concern:
.jpg)
This end results can also be fused with imagery, giving you further confidence in the accuracy of the location determined of your assets, as well as providing a unique perspective of the corridor for planning:
Though this specific use case focused on identifying the assets along a rail corridor, the same LiDAR dataset can be used to run additional analytics like automatically extracting your rail lines through NV5's Automated Rail Extraction tool, determining vegetation hazards near these lines, and modeling elevation and slope along the rail corridor.
As the sensors continue to evolve in their size and flexibility, this maturing data source will allow Rail Roads to get unique perspectives that allow them to not only get a more accurate count on assets as well as run predictive analytics to help reduce maintenance costs.