15 Nov 2016 08:28 AM |
|
Hello ENVI users and Admins,
I'm looking for an easy to follow tutorial for using ENVI to perform linear spectral unmixing on a LANDSAT7 ETM+ image.
I'm relatively new to this process (MS student) and having a difficult time getting any meaningful results from my analysis, using a V-I-S classification system.
My process thus far includes basic data prep in ArcMap 10.3 (clipping and projecting), digitizing a ground truth image based on random points and a 3x3 pixel (90x90m) square, based on LandSat7 pixels.
In ENVI, I've used the MNF feature to reduce the dimensionality of the data, going from six bands to four. From there, I've added ROIs in a V-I-S theme, using the ROI tool to pick out pixels individually. I then run the Linear Spectral Unmixing tool off of the ROIs from this MNF-reduced file. Convert to a TIFF, bring it into ArcMap, and compare to the digitized ground-truth images via Z-tables. Thus far, the results aren't great. However, I should note that I'm only using 50 or so pixels for each type of material.
Alternatively, I've thought about using the scatter plots of the MNF results bands. Compared to individual pixel selection and building ROIs through the ROI tool, it seems like you'd need to spend an inordinate amount of time making sure that the pixels you select in the scatter plots are actually the material (V-I-S) in question.
What is an appropriate sample number? Should you use multiple MNF plots (MNF 1 vs. 3, MNF 2 vs. 3, etc?)? Does this help guarantee representative sampling to generate ROIs for feeding into the Linear Spectral Unmixing Tool?
Thanks for the help!
-Andy
|
|
|
|
MariM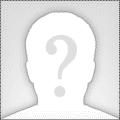 Veteran Member
Posts:2396  
15 Nov 2016 01:21 PM |
|
Have you tried using the Spectral Hourglass wizard?
http://www.harrisgeospati...HourglassWizard.html
This walks you through the process of reducing data dimensionality so that you can find image endmembers for unmixing the image. It describes each step along the way.
One issue with using data such as ETM is that you have relatively few bands for finding endmembers while unmixing relies on the ability to find all the unique materials (endmembers) in your scene to calculate abundance. It works best with over-sampled data (hyperspectral) images because you can only have nb-1 unique materials in the image. For a 7-band image, that would mean 6 endmembers. However, the wizard also has other options for output such as MTMF, where you find specific endmembers compared to all others (the background). You might find this to be a better approach but definitely try all the available methods. The Wizard also has an automatic extraction of endmembers from the data based on statistics so it is less cumbersome than searching the n-D visualizer manually (but the results should also be checked!). You will also need to find out what materials the extracted endmembers represent. This can be done manually (perhaps they are easily identified in the image) or with comparison to a spectral library of known materials in the image.
There is no specific number of pixels that should be selected as a representative (endmember) sample. A single outlier pixel may represent a rare material in the image. A small cluster on the edges of the cloud probably represent another material. As you load up different MNF images, you may see the cloud change shape so you look for clusters or outliers that remain clustered and compare them to known materials in the image. Compare them to the statistically extracted endmembers and adjust the selected pixels as needed.
|
|
|
|
Deleted User New Member
Posts:  
01 Dec 2016 04:57 PM |
|
Mari,
I have a follow up question, and as it's on the same subject I'll just ask it here.
I'm using the Scatter Plot tool to (using MNF reduced data, from 6 bands to 4) to generate ROIs. I'm using MNF4 and MNF3, but would also like to use the comparison of MNF4 and MNF2 to make additional ROIs.
I know that I can make more ROIs from the second scatter plot, but will I be able to use them in Linear Spectral Unmixing later on? Meaning, can I generate two ROI files, from two scatter plots (each with the same four classes), and use both of them as inputs?
|
|
|
|
MariM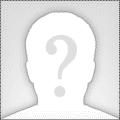 Veteran Member
Posts:2396  
02 Dec 2016 01:40 PM |
|
I don't see why not. I was able to open an MNF image and some previous ROIs I had created on the image (3). I then created a scatterplot, created some different ROIs using different MNF band combinations and exported those selections to new ROIs. These ROIs were automatically added to the current set of ROIs I had open. When I opened the LSU tool and selected to import ROIs, the dialog was aware of all of the ROIs (old and new).
I am doing this in ENVI 5.3.1 (not Classic).
|
|
|
|
Deleted User New Member
Posts:  
03 Dec 2016 06:34 PM |
|
Mari,
I just tried using the Spectral Hourglass Wizard.
Loaded my water-masked LandSat scene as the input, specified an output file name. Started going through the steps. Selected "4" as my number of output bands from the MNF transformation. Click next, generate MNF-transformed scene.
Next, I selected "yes" when prompted to derive endmembers from the image.
Kept the defaults for the PPI interations, PPI threshold value, and PP maximum memory to be used.
At examine PPI results step, kept the default 10,000 pixels to be used in the n-D visualizer.
I hit the "next" arrow, and then I get this message: "SVDC: Argument A must be a 2-D matrix: MEANS". When I hit OK, the program crashes.
Is there anything I can do to fix this?
|
|
|
|
Deleted User New Member
Posts:  
03 Dec 2016 09:39 PM |
|
I've also tried selecting pixels from the scatter plots of the MNF-transformed data. At the moment, the best I've done for correlation is an r2 of 0.64. Not exactly knocking it out of the park.
Comparing my random 90x90m digitized ground truth squares to the 2011 NLCD impervious surface layer, they have an r2 of 0.80.
Here is an image of the scatter plot of the pixels in the image, using MNF-transformed Band 3 and Band 4. Again, this is the best I've done, at r2 = 0.64. I used it generate ROIs, which were then used as inputs in the Linear Spectral Unmixing tool.
https://drive.google.com/open?id=0B-qDNCi6oOLIcUxseG9OYzIyRkk
I feel like I must be doing something very, very wrong.
|
|
|
|
MariM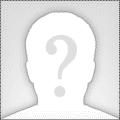 Veteran Member
Posts:2396  
05 Dec 2016 12:47 PM |
|
I am not sure why you would get this error at this point but I wonder if you might try a couple things:
- do not spectrally subset the MNF bands - you have relatively few bands and it is most likely they all contain useful information.
- you mentioned you used masked data. Did you apply the mask to the data prior to using it in the Wizard? Or did you select a mask band when you selected your input file (there is a file selection dialog that allows you to also select a mask band). I would be sure to select a mask band on input to exclude pixels.
You mentioned that you might also be selecting ROIs outside of the wizard. I assume you have not yet added these yet and are only trying to generate endmembers using the data and the wizard method. Is this correct? Can you complete the wizard using only this method?
Did you calibrate your data to TOA or surface reflectance?
|
|
|
|
Deleted User New Member
Posts:  
06 Dec 2016 04:30 PM |
|
I have not calibrated my reflectance.
At this point, I'm ONLY two files, a 6-band LandSat image plus a raster file of sand/water I wish to mask out. I have used the Layer Stacking function to create one 7-layer stack, with the mask integrated as the 7th band.
From there, I import that one layer into the Spectral Hourglass Wizard, select the mask band accordingly, and let it run.
Keep getting the same error. I'm about to head to school to see if the computers in my lab have the same problem.
|
|
|
|
MariM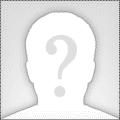 Veteran Member
Posts:2396  
07 Dec 2016 07:22 AM |
|
When you select the file for input, to you do a spectral subset to exclude the mask as input and then select that band for the mask? They should be kept separate.
What if you just input the Landsat file without the mask? It might help us narrow down if it is a problem with masking.
|
|
|
|
Deleted User New Member
Posts:  
13 Dec 2016 02:16 PM |
|
Ok. Got it to work with the 7-layer, mask-included file.
Not sure what changed. Tried restarting ENVI quite a few times, then restarted my computer and it works now.
Now, the problem I'm having is that I don't understand how to use the n-D visualizer to select endmembers. When I was selecting ROIs using the scatter plots previously, I could view the ROIs by placing that layer on top of an NAIP or DOQQ file. Now, the automatic clustering method that ENVI uses to group the pixels doesn't really show me much. I can't tell how the pixels are being grouped, other than they appear clustered about the same point in n-dimensional space. Put another way, I have no clue what materials those clusters are supposed to represent.
Do you offer any classes or hands on demonstrations at your Boulder headquarters? I'm going to be in Fort Collins for our month-long winter break and really, really need to get a handle on this.
|
|
|
|
Deleted User New Member
Posts:  
14 Dec 2016 03:32 PM |
|
Mari,
I've searched and I've played around with the n-D visualizer to select endmembers, having reduced my data set from 6 bands to 4. No one I know knows how to use this function. Can you recommend ANY source of help for me?
Thanks.
|
|
|
|
MariM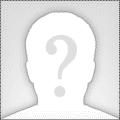 Veteran Member
Posts:2396  
16 Dec 2016 11:10 AM |
|
The online help provides some descriptions on how the nD visualizer works and the tutorials can show you "how" to use it:
http://www.harrisgeospatial.com/docs/...
But it does have a learning curve to understand the MNF images, what the PPI results are showing and why the visualizer clusters pixels in the way it does (by pixel counts from the PPI). The idea is that the endmembers (purest pixels and unique materials) are found at the edges of the convex hull of the n-d pixel cloud. The pre-cluster results are finding those pixels from the statistics of the PPI results. It will tell you nothing of the nature of the pixels it finds (what material it might be) so it is up to the analyst to either examine the ROIs selected from the nD visualizer on the original image to determine the material, or if you have a spectral library, you can compare the exported spectral endmembers to the library to determine what material it is.
The problem may be that what you are trying to find (e.g. multiple vegetation types) are not spectrally distinguishable using multispectral data.
You might contact our training department about when and where there will be training events you can attend:
http://www.harrisgeospatial.com/Learn...
|
|
|
|
Deleted User New Member
Posts:  
22 Dec 2016 03:02 PM |
|
Mari,
I emailed the Geospatial Training department at the address listed on your website (geospatialtraining@harris.com) on the 17th. Haven't heard back yet.
To address one of your previous concerns: I don't need to distinguish between various vegetation types. At the end, I simply need to get an estimation of the percentage of each pixel that is made up of vegetation, impervious surface, or soil (V-I-S). This is a model that was developed by Kidd, M. K. (1995) and has been explored in depth over the years. Notably, Wu and Murray (2003) used this model in a very well done experiment using Columbus, OH as a study area.
Basically, each pixel a given study area is assumed to be made up of a combination of V, I or S. That is, 1 = V+I+S. By using linear spectral unmixing, they were able to get an R2 of 0.85. I'm attempting to do something similar with a different study area.
I'm still struggling with defining the endmembers. I don't know where to go from here.
Thanks,
Andy
|
|
|
|
Deleted User Basic Member
Posts:228  
11 Jan 2017 08:22 AM |
|
Dear Andy,
Our training department is in the middle of changing systems for organizing and presenting our courses, so the training class information is not described well on the website at this point. I have forwarded your request for information about training to our training coordinator and asked her to get back to you ASAP with information about our upcoming ENVI spectral analysis trainings.
Regarding your confusion around how to pick endmembers, it is indeed a tricky thing that is hard to learn. And there are multiple ways you can go about it. I'll try to describe the most common here, as best I can.
First, lets talk about preprocessing: It sounds like you have created a mask image for water and bright sand/soil, and you are choosing it as the "mask band" when you pick your input file for the linear spectral unmixing. That's a good start. Also definitely make sure that your mask band is not included as one of the bands in the input file that is being used for the unmixing. Mari referred to that earlier, and you didn't comment on whether you were choosing a spectral subset of only the true Landsat bands when importing your layer stack. You will absolutely need to do that if you've made a layer stack with the mask band as one of the layers. That mask band must not be considered an image band during the unmixing. ENVI doesn't automatically know what is a real band and what is a mask. It assumes everything in the layer stack image is a real band, unless you explicitly tell it to leave out that one mask band.
It also sounds like you have declined to perform radiometric calibration and atmospheric correction. Which involves some assumptions. Most importantly, you are assuming that the presence of the atmosphere is not varying over the scene. If that's not the case in your area (for example, if it's a humid area), then that will affect the accuracy of your results. Just be aware of that.
Since there is no atmospheric correction, you will need your endmember spectra to be derived from actual pixels in the image. Those pixel spectra will also have the same atmospheric and solar conditions. Any endmember spectra derived from separate measurements (e.g., collected with a field spectrometer or a lab spectrometer) will look very different for the same materials than those materials will look in the form of pixel spectra from your image.
So, knowing that you need pixel spectra as your endmembers, there are a couple of ways to find those. If you know where there are good pure examples of the endmembers in question in your scene, you can just create (draw) ROIs that includes those pixels. Then the spectra from those ROIs can be used as endmembers. The problem with doing this is that you can't be sure that the pixels you chose to represent your endmember spectra are the *best* (as in most pure) examples of your endmember spectra. You're just making that assumption. If you are very sure that this assumption is correct, then you can certainly proceed from here with linear spectral unmixing using those ROIs, and avoid the complication of the rest of the hourglass workflow. But if there happen to be other pixels in the scene that are more pure, then the unmixing results you get for those pixels will not make much sense. For example, they may look like they contain more than 100% of one endmember, and less than 0% of others. Or the RMS error for the unmixing analysis will show distinct patterns related to real features on the surface, instead of just looking like noise.
The hourglass processing workflow in ENVI was designed to address that potential problem. The idea there is that you are finding the purest pixels in your scene (i.e., the ones that really contain 100% one material). The MNF step helps you reduce the dimensionality of your data to its true dimensionality. In that step, it sounds like you are choosing to end up with 4 bands, because you go into the analysis assuming that there are 4 distinct materials in the scene: vegetation, soil, bright impervious surfaces, and dark impervious surfaces. But there is no guarantee that that is actually true. You may have some other materials in there that are spectrally distinct, and mixing with those other materials. That is why Mari recommended to you that you don't reduce the data to only 4 MNF bands. If you leave all 6, then that will give you a chance to evaluate your assumption that there are only 4 spectrally distinct materials in your scene. If you force ENVI to consider only the first 4 MNF bands, there is no guarantee that those describe the four materials you are assuming are most spectrally influential in your scene. Maybe there is something else out there that accounts for one of those MNF dimensions, and one of the materials you're assuming is there is actually best represented in MNF band 5 or 6.
OK, so the next step then is the PPI step. This is designed to find only the purest pixels in your scene. We do this because the following step, with the n-D visualizer can't work with all of the pixels in a typical image. And really, you don't care about all of the pixels that fall in the center of the data cloud. Those are obviously not endmembers. They are mixed pixels.
Once you've created your big ROI from the PPI step, which contains all of the pure pixels of whatever materials, you then import that into the nD Visualizer. This is a confusing tool, but it's the best way we've found to accomplish the task it's designed to do. Which is to identify which of the pure pixels are the best examples of the different real spectral endmembers (spectrally distinct materials) in the scene. This step doesn't tell you which of the ROIs you'll be creating are which materials. That comes later in a different step.
Using the nD Visualizer is kind of like what you've been trying to do with the scatter plots of the different MNF bands. Only it lets you see the data cloud in more than 2 dimensions at a time. The problem with what you're doing with the scatter plots is that you may be missing some corners of the data cloud that don't show up in a 2D scatter plot of only 2 MNF bands. Maybe some corner is like that tetrahedral corner that is pointing right out at you, and looks like it's in the middle of the data cloud. So you'd totally miss that endmember. Instead, the nD Visualizer lets you view the data cloud in all of the dimensions there are (in your case, that's 6). It rotates, so that if a corner is hidden in one rotation, it will eventually pop out in a different rotation. Your job is to find where those corners pop out, and circle the pixels in the most extreme ends of that corner in a separate color.
If your assumption that only vegetation, soil and bright and dark impervious surfaces are spectrally significant is true, then you should see four obvious corners pop out. If you see more obvious corners than that, then you've got some other material(s) out there, and your assumption is not correct.
So, lets assume that you see four obvious corners, and you circle them in different colors in the nD Visualizer, and then you export those to ROIs. Now you have four ROIs for your image. You can display those ROIs on the image to see if that tells you which ROI is which material. If you can't tell by context, then you can try comparing the spectra from those ROIs to a spectral library of known material spectra. But that's going to be tricky in your case, because your image spectra are not radiometrically calibrated and atmospherically corrected. So they won't look like reflectance spectra. Hopefully context will help you know which is which.
Now you're ready to perform linear spectra unmixing, with your four (hopefully) ROIs as endmembers. If you ended up with more than 4 ROIs (more than 4 obvious corners in the nD Visualizer), then you'll want to use all of those endmembers in the unmixing. It doesn't work to ignore spectrally significant endmembers. You just end up with bad (wrong, and/or nonsensical) fractions of your endmembers in each pixel. An important assumption of the linear spectral unmixing model is that you've accounted for all spectrally distinct materials in the scene.
I hope this information helps. If you still have questions, can you please be specific about exactly what those questions are? I'll be happy to try to help clarify more.
Best,
Peg
|
|
|
|
Deleted User Basic Member
Posts:228  
11 Jan 2017 01:18 PM |
|
Dear Andy,
I took a look at your screen shots, and it looks like you are setting up the hourglass workflow correctly, in terms of which bands to choose and how to specify your mask band. I didn't see a screen shot of what your mask looks like, so I can't comment on that.
I explained in my last message why I would recommend using all 6 MNF bands after the MNF transform step. But if you are following a process used by others in which they did not do so, and if your situation (data, scene components, etc.) is similar enough to those people's that the same assumptions apply, and you agree with their assumptions, you could use a different spectral subset. ENVI certainly allows for that possibility.
Regarding having you stop by for one-on-one coaching for your spectral processing in ENVI, I am afraid that we are not able to offer that service along with your ENVI license. If you are interested in purchasing that type of coaching, I can put you in touch with your sales representative, and let them know that your time frame is very short to set this up while you are still in Colorado. Just let me know.
Regards,
Peg
|
|
|
|
Deleted User New Member
Posts:  
11 Jan 2017 01:26 PM |
|
Peg,
Yes, I would be interested in being put in touch with your sales representative. Unfortunately, I have to leave to head back to VA on Saturday, so the 12th and 13th are the only days that would work for me.
-Andy
|
|
|
|
Deleted User Basic Member
Posts:228  
11 Jan 2017 01:51 PM |
|
Dear Andy,
I have contacted your sales rep. I am not sure whether we could get this set up within your time frame. I will let you know as soon as I hear back with any additional information.
Regards,
Peg
|
|
|
|