18684
ENVI Deep Learning training error: CUDNN_STATUS_ALLOC_FAILED
What is the cause of the error 'CUDNN_STATUS_ALLOC_FAILED' when training a model using the ENVI Deep Learning module?
A message that references "CUDNN_STATUS_ALLOC_FAILED" or a "ResourceExhaustedError" is a GPU memory allocation error that often occurs when the graphics card or drivers are not up to specification requirements.

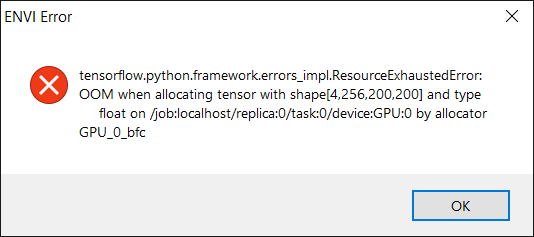
CUDNN memory allocation error and ResourceExhaustedError
ENVI Deep Learning leverages TensorFlow™ technology to train deep learning models within the ENVI user interface. The underlying TensorFlow deep learning code is optimized for NVIDIA GPUs. GPU acceleration is only supported on NVIDIA GPU cards with CUDA® Compute Capability 3.5 or higher. You can see a list of CUDA-enabled GPU cards at https://developer.nvidia.com/cuda-gpus.
WIth ENVI Deep Learning, we recommend using a GPU with at least 8 GB of memory and it must meet the NVIDIA® driver version requirements (384.x or higher). While you can run the Deep Learning module with a CPU rather than GPU, it is not recommended because the deep learning training process is computationally intensive and you will greatly benefit from the use of a dedicated GPU.
If you encounter a memory allocation error in Deep Learning, there are a couple of settings you can try to resolve the error and allow the training to continue.
- First try to reduce the Patch_Size parameter when initializing a new model. The default value is 572, try a much smaller value and see what works.
- Adjust the Number of Patches per Batch, in the Train task. It is usually best to leave it at the default and let ENVI decide what value to use, but in cases where memory errors persist, it may help to set the value to 2 to begin with, then increase if possible to make training faster.
For more information on ENVI Deep Learning please see the FAQ.
Created 5/20/2019 - mm
Reviewed 5/21/2019 - e.iturrate