Advanced GEOINT Series Blog 2 : Streamline Data Labeling and Model Training
For geospatial intelligence to be effective, it must be efficient. The ENVI® Ecosystem combines advanced solutions that streamline complex GEOINT workflows, making it easier for organizations to capture, analyze, and act on data quickly.
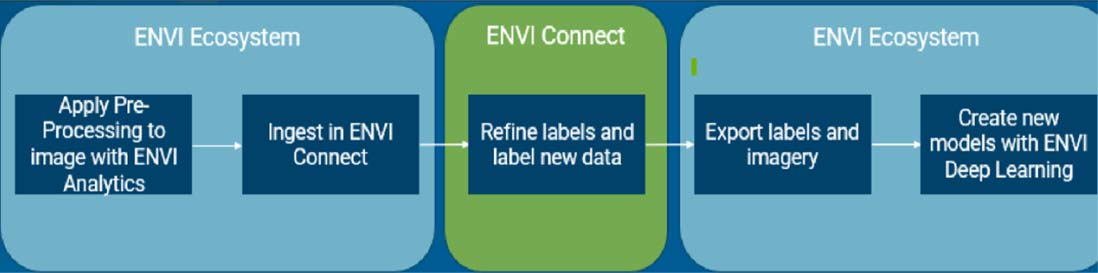
Figure 1 illustrates the end-to-end ENVI Ecosystem approach for creating labeled data and Deep Learning object detection models.
This blog will focus on the process of creating accurate training data and developing reliable models, which is often labor-intensive, especially in geospatial analysis. The ENVI Ecosystem streamlines the entire workflow – from data labeling to model training – so users can quickly identify features of interest and generate actionable insights.
With ENVI Connect (one tool within the ENVI Ecosystem), it’s easy to produce labeled data at scale – up to 800 objects per hour. With an intuitive, streamlined interface, ENVI Connect enables users to mark and label objects in images, creating structured data sets that serve as the foundation for training machine and deep learning models.
Why does this matter? Labeling specific objects allows models to differentiate between different features, improving detection accuracy and reducing false positives. The labeling capability within ENVI Connect ensures that AI models can be highly specialized and tuned for specific tasks.
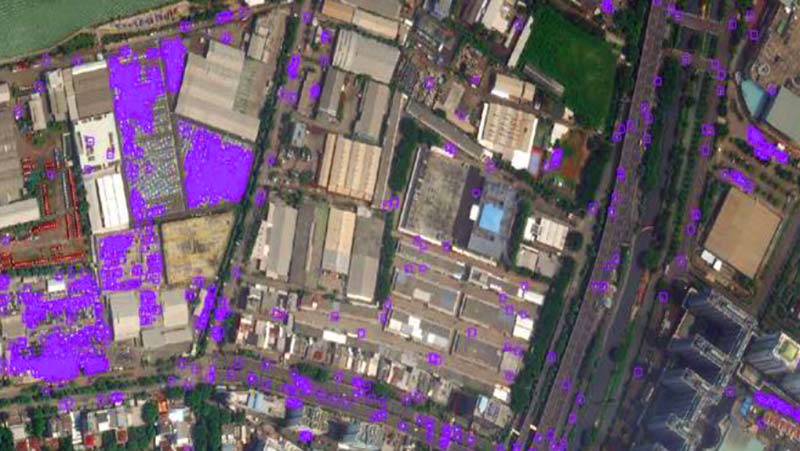
Figure 2 shows the results of applying the classifier to an image. SUVs were detected throughout the image.
Once data has been labeled, the next step is training a model. ENVI leverages open-source tools like TensorFlow to give users a robust training environment. By automating data retrieval from ENVI Connect, ENVI simplifies the process of loading training data and starting the model training process. Whether you’re working with object detection, segmentation, or custom grid models, ENVI makes it easy to build models tailored to your needs.
ENVI runs on both Windows and Linux, making it accessible across different setups. This flexibility also extends to its hardware requirements: with support for GPU-accelerated training, allowing teams to process large datasets quickly, leading to faster insights and quicker deployment.
-
Rapid Data Labeling: Label hundreds of objects per hour for faster model development.
-
Import Labels as Needed: Labels can be imported from GeoJSON or Shapefiles from open-source libraries or other systems.
- Select Labels Efficiently: Multiple staff members can simultaneously select labels for objects.
The ENVI Ecosystem streamlines the often-complex processes of data labeling and model training, empowering teams to quickly develop high-performing models that support critical geospatial insights.
Our experts are happy to discuss how a solution like this can support your mission – email us.
Advanced GEOINT Blog Series